Transforming Supply Chains with AI: A Strategic Approach
- Raul Porri
- Feb 27
- 6 min read
This guide outlines a strategic framework for integrating AI into your supply chain, supported by real-world examples, data-driven insights, and actionable recommendations. Whether you’re looking to improve demand forecasting, streamline logistics, or enhance supplier relationships, this approach will help you harness the full potential of AI to drive measurable business outcomes.
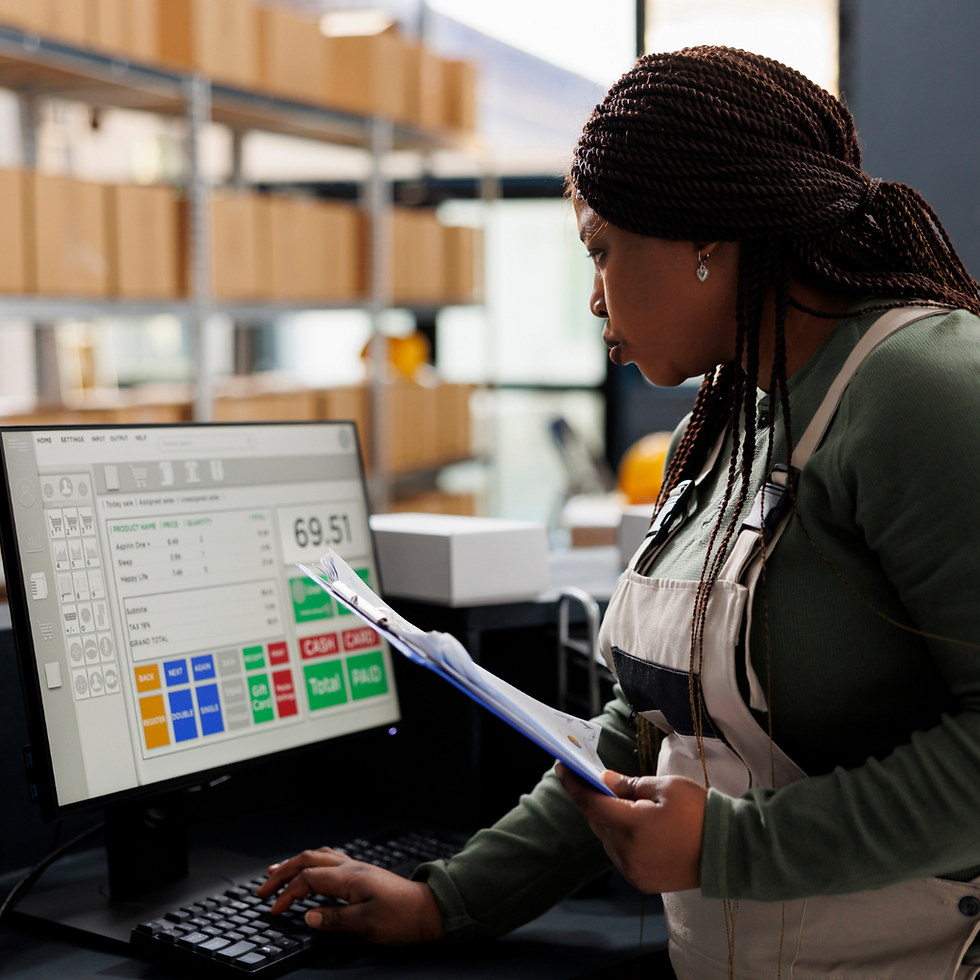
1. Assess Your Supply Chain Needs
Before implementing AI, identify the pain points in your supply chain. Common challenges include:
Inaccurate demand forecasting
Inefficient inventory management
Delays in logistics and delivery
Example: A retail company struggling with overstocking and stockouts used AI to analyze historical sales data and predict future demand more accurately.
Common Supply Chain Challenges and AI Solutions
Challenge | AI Solution |
Demand Forecasting | Predictive analytics |
Inventory Management | AI-powered inventory optimization |
Logistics Optimization | Route optimization algorithms |
Supplier Risk Management | AI-driven risk assessment tools |
2. Choose the Right AI Technologies
Select AI tools that align with your supply chain goals. Key technologies include:
Machine Learning (ML): For predictive analytics and demand forecasting.
Natural Language Processing (NLP): For analyzing supplier contracts and customer feedback.
Computer Vision: For automating quality checks in warehouses.
Example: Amazon uses computer vision and robotics in its warehouses to automate sorting and packing, reducing processing time by 25%.
AI Technologies and Their Applications
Technology | Application |
Machine Learning | Demand forecasting, inventory management |
Natural Language Processing | Supplier contract analysis |
Computer Vision | Quality control, warehouse automation |
Robotics | Picking, packing, and sorting |
3. Integrate AI with Existing Systems
Ensure your AI tools integrate seamlessly with your current supply chain management systems (e.g., ERP, WMS).
Example: Walmart integrated AI with its ERP system to optimize inventory levels across 4,700 stores, reducing excess stock by 10%.
Actionable Tips:
Use APIs to connect AI tools with existing platforms.
Ensure data compatibility and standardization.
4. Collect and Clean Data
AI relies on high-quality data. Collect data from all supply chain touchpoints, including suppliers, warehouses, and customers. Clean the data to remove inconsistencies and errors.
Example: A global manufacturer used AI to analyze supplier performance data, reducing lead times by 15%.
Key Data Sources for AI in Supply Chain
Data Source | Use Case |
Sales Data | Demand forecasting |
GPS and IoT Sensors | Logistics optimization |
Supplier Performance Data | Risk assessment and supplier selection |
Customer Feedback | Demand planning and product improvement |
5. Start with Pilot Projects
Implement AI in a small, controlled environment to test its effectiveness.
Example: A logistics company piloted an AI-powered route optimization tool for a single delivery route, reducing fuel costs by 12%.
Actionable Tips:
Choose a high-impact area for your pilot.
Measure KPIs such as cost savings, delivery times, and accuracy.
6. Scale and Optimize
Once the pilot is successful, scale the AI solution across your supply chain. Continuously monitor performance and optimize the system.
Example: Unilever scaled its AI-powered demand forecasting system globally, improving forecast accuracy by 20%.
Data Table: KPIs to Measure AI Success
KPI | Target |
Forecast Accuracy | 90%+ |
Inventory Turnover Ratio | Increase by 15-20% |
Delivery Time | Reduce by 10-15% |
Cost Savings | 10-20% reduction in operational costs |
7. Train Your Team
Equip your team with the skills to use AI tools effectively. Provide training on data analysis, AI tools, and change management.
Example: A pharmaceutical company trained its supply chain team on AI tools, reducing manual errors by 30%.
Real-World Success Stories
DHL: Used AI to optimize delivery routes, reducing fuel consumption by 15%.
Procter & Gamble: Implemented AI for demand forecasting, cutting inventory costs by 20%.
Maersk: Leveraged AI for predictive maintenance, reducing downtime by 25%.
We explored the foundational steps for implementing AI in your supply chain, from assessing needs to scaling solutions. Now, let’s dive deeper into advanced strategies, emerging trends, and practical tips to ensure your AI implementation delivers maximum value. We’ll also address common challenges and provide actionable advice for overcoming them.
Advanced Strategies for AI Implementation
1. Leverage AI for End-to-End Visibility
End-to-end visibility is critical for supply chain efficiency. AI can integrate data from suppliers, manufacturers, distributors, and customers to provide real-time insights.
Use Case: Track shipments in real-time using IoT sensors and AI analytics.
Example: FedEx uses AI-powered tracking systems to provide customers with real-time updates on package locations.
Benefits of End-to-End Visibility
Benefit | Impact |
Reduced Delays | 20-30% improvement in on-time delivery |
Improved Customer Satisfaction | 15-25% increase in customer retention |
Enhanced Decision-Making | Faster response to disruptions |
2. Adopt Predictive Maintenance
Predictive maintenance uses AI to monitor equipment and predict failures before they occur, reducing downtime and maintenance costs.
Use Case: Monitor machinery in manufacturing plants using IoT sensors and AI algorithms.
Example: General Electric (GE) uses predictive maintenance to reduce unplanned downtime by 20%.
Predictive Maintenance ROI
Metric | Improvement |
Downtime Reduction | 20-30% |
Maintenance Cost Savings | 10-15% |
Equipment Lifespan | Extended by 15-20% |
3. Optimize Supplier Relationships with AI
AI can analyze supplier performance data to identify risks, improve collaboration, and ensure timely deliveries.
Use Case: Use AI to evaluate supplier reliability and negotiate better contracts.
Example: Walmart uses AI to assess supplier performance, reducing lead times by 15%.
Supplier Optimization Metrics
Metric | Impact |
Lead Time Reduction | 10-20% |
Supplier Risk Mitigation | 25-30% improvement in risk assessment |
Cost Savings | 5-10% reduction in procurement costs |
Emerging Trends in AI for Supply Chains
1. AI-Powered Digital Twins
Digital twins are virtual replicas of physical supply chains. AI-powered digital twins enable real-time simulation and optimization.
Use Case: Simulate supply chain scenarios to identify bottlenecks and test solutions.
Example: Siemens uses digital twins to optimize production processes, reducing downtime by 50%.
2. Autonomous Vehicles and Drones
Autonomous vehicles and drones are transforming logistics, enabling faster and more cost-effective deliveries.
Use Case: Use drones for last-mile delivery in remote areas.
Example: Amazon Prime Air uses drones to deliver packages in under 30 minutes.
3. Blockchain for Supply Chain Transparency
Blockchain, combined with AI, enhances transparency and traceability in supply chains.
Use Case: Track the origin of raw materials and ensure compliance with regulations.
Example: IBM Food Trust uses blockchain and AI to track food supply chains, reducing fraud and improving safety.
Practical Tips for Overcoming Challenges
Implementing AI in your supply chain is not without challenges. Here’s how to address common obstacles:
1. Data Quality Issues
Poor-quality data can undermine AI effectiveness.
Solution: Invest in data cleaning tools and establish data governance policies.
2. High Implementation Costs
AI implementation can be expensive, especially for small and medium-sized enterprises (SMEs).
Solution: Start with low-cost pilot projects and scale gradually.
3. Resistance to Change
Employees may resist adopting AI tools.
Solution: Provide training and communicate the benefits of AI.
4. Integration with Legacy Systems
Legacy systems may not be compatible with AI technologies.
Solution: Use middleware or APIs to bridge the gap between old and new systems.
Measuring the Success of Your AI Implementation
To ensure your AI implementation delivers value, track key performance indicators (KPIs). Here’s a comprehensive list of KPIs to monitor:
AI Supply Chain KPIs
KPI | Target |
Forecast Accuracy | 90%+ |
Inventory Turnover Ratio | Increase by 15-20% |
Delivery Time | Reduce by 10-15% |
Cost Savings | 10-20% reduction in operational costs |
Supplier On-Time Delivery | 95%+ |
Customer Satisfaction | 20-25% improvement |
Real-World Success Stories
4. Nestlé: AI for Demand Forecasting
Nestlé implemented AI to analyze sales data and predict demand more accurately, reducing excess inventory by 15%.
5. Zara: AI for Inventory Optimization
Zara uses AI to optimize inventory levels across its global stores, reducing stockouts by 20%.
6. UPS: AI for Route Optimization
UPS uses AI-powered route optimization tools to save 10 million gallons of fuel annually.
Conclusion: Transform Your Supply Chain with AI
AI is a game-changer for supply chain management, offering unprecedented levels of efficiency, accuracy, and cost savings. By following this step-by-step guide, you can successfully implement AI in your supply chain and stay ahead of the competition.
Key Takeaways:
Start by identifying your supply chain pain points.
Choose the right AI technologies for your needs.
Pilot, scale, and optimize your AI solutions.
Train your team to maximize the benefits of AI.
Leverage AI for end-to-end visibility and predictive maintenance.
Optimize supplier relationships and adopt emerging trends like digital twins and blockchain.
Overcome challenges by focusing on data quality, cost management, and change adoption.
Measure success using KPIs such as forecast accuracy, delivery times, and cost savings.
Ready to transform your supply chain with AI? Contact us.